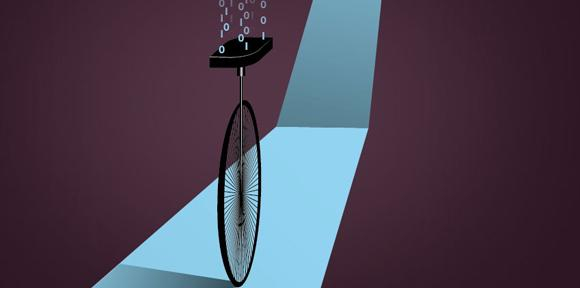
Cambridge researchers have pioneered a robot unicycle that can learn on it own, falling for the first time but learning how to stay stable longer next time, just like any human kid. The AI experiment has huge potential ranging from self-driving cars to credit card fraud.
The incremental learning model is based on the 18th century mathematical model of English statistician Thomas Bayes known as Bayesian probability theory that describes how the probability of an event occurring is updated as more evidence becomes available.
Shown in an animation, the unicycle lurches forward and falls on its first trial. But on its second trial, there is a perceptible change, though it was just a few more seconds of delay in the fall. The robot, aided by artificial intelligence, is actually trying to correct itself before the inevitable fall. It took just one minute to learn the trick and was seen gently rocking back and forth and circling on the spot, maintaining an extremely stable system.
"It's learning from experience," explained Professor Carl Edward Rasmussen, who leads the Computational and Biological Learning Lab in the Department of Engineering, University of Cambridge. "The unicycle starts with knowing nothing about what's going on – it's only been told that its goal is to stay in the center in an upright fashion. As it starts falling forwards and backward, it starts to learn," said Rasmussen.
The machine can self-learn and improve its knowledge every time it gets new information. "This is just like a human would learn," said another researcher Zoubin Ghahramani, who leads the Machine Learning Group in the same department. "We don't start knowing everything. We learn things incrementally, from only a few examples, and we know when we are not yet confident in our understanding."
Ghahramani's team is working on the incremental machine learning based on neural networks and deep learning models using complex algorithms to make them helpful in day-to-day applications such as translating phrases into different languages, recognizing people and objects in images, and detecting unusual spending on credit cards, besides embedding them on driverless cars.
Pointing out the defect in most of the current AI models which are confined to data already fed, he said the outcome was often dismal."When you test them outside of the data they were trained on, they tend to perform poorly and sometimes provide confidently wrong answers. This is what bothers me. It's okay to be wrong but it's not okay to be confidently wrong," said Ghahramani.
"Driverless cars, for instance, may be trained on a huge dataset of images but they might not be able to generalize to foggy conditions."
Ghahramani, also Chief Scientist at Uber, sees immense potential of making driverless cars learn not just individually but as part of a group. "Whether it's companies like Uber optimizing supply and demand, or autonomous vehicles alerting each other to what's ahead on the road, or robots working together to lift a heavy load – cooperation, and sometimes competition, in AI will help solve problems across a huge range of industries," he said.
One of the really exciting frontiers is being able to model probable outcomes in the future, as Turner describes. "The role of uncertainty becomes very clear when we start to talk about forecasting future problems such as climate change." Some scientists believe the self-learning techniques can improve the forecast of future climate change risks.