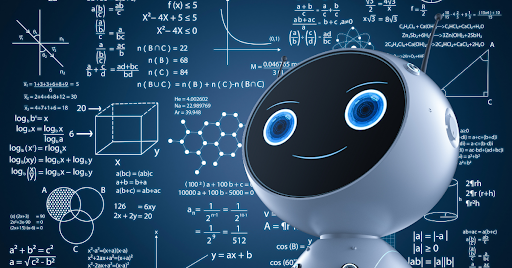
Artificial intelligence (AI) is one of the four most powerful general-purpose technologies to impact the world in the last two centuries. It is stirring business change, just like information technology has done since the 80s. Like the steam engine and electricity, machine learning and AI inspire billion-dollar complementary innovations.
As per a Brookings study, the entity that leads in AI innovation by 2030 could be a world leader until 2100. Unlike special-purpose technologies like landline phones, which are easily replaceable with mobile phones, no business can leapfrog over AI use.
Every business must advance in AI for relevance and sustainability. But unfortunately, as AI disruption and transformation registers in the world's economies, there is rising alarm about the risks inherent in AI development.
Widespread AI use will affect how populations approach and engage critical topics such as rights, values, and religion. But unfortunately, only a handful of people decide on the policies that guide the AI approach to social, political, and cultural topics.
Prominent AI engineers say that building explainable AI can alleviate many of these fears. In addition, explainable AI methods and processes can help data science leaders and machine learning engineers comprehend the output that machine learning algorithms generate.
More than that, explainable AI processes can help human users trust an AI model's results. AI explainability places accuracy, ethics, transparency, and trust at the heart of AI model production.
The Qwak explainable AI solution
Qwak is a fully managed machine learning platform that provides a superior explainable AI development infrastructure to data science leaders and machine learning engineers. Qwak tools such as its Build System, Model Serving, and Data Inference Lake help data scientists and engineers explain how their AI algorithms arrive at specific results.
The Qwak Build System, for instance, effortlessly transforms machine learning code into production-grade AI solutions. The beauty of this feature is that it standardizes all machine learning project development by automatically versioning code, data, and model build parameters.
Its standardization, remote build, and version management tools support AI model comparability. In addition, these tools facilitate model generation on remote elastic resources. So, you can, for instance, build deployable and reusable models free of the AI black box problem.
Consequently, Qwak's reusable past builds are easy to explain and understand. As a result, data science leaders and machine learning engineers will have an easy time identifying and correcting biases in their algorithms.
Qwak's Model Serving feature, on the other hand, supports the management deployments and the serving of AI models at scale. This feature lowers friction between AI engineers and their data science counterparts and generates repeatable explainable AI models.
Model Serving tools such as observability tracks AI model logs, performance, and other metrics, delivering predictions in a repeatable way. The Qwak Inference Lake offers data science leaders and machine learning engineers an easy-to-use and accessible data collection, storage, and analysis system.
Teams can easily analyze model data and access all feedback, inferences, and model baseline data. The Qwak Inference Lake's other benefits are effortless model performance management, audit, and training observability.
Create explainable AI using Qwak tools and features and offer users inclusive and interpretable machine learning models that detect and remedy model errors and bias. Qwak will ensure that you confidently deploy your models and enjoy high performance and scalability metrics.
The prominent AI engineers behind Qwak's explainable AI processes include CEO Alon Lev, a former VP of Data & Site manager at Payoneer. Yuval Fernbach, the Qwak CTO, has worked as the ML Specialist (Sagemaker) for AWS EMEA. In addition, Ran Romano, the platform's VP of Engineering, has led the Data & ML Engineering groups at Wix.com.