The progression of neurodegenerative diseases is not the same among all patients. Therefore, providing effective treatment is a challenge owing to the diversity in the individual progression of these diseases. However, a new study claims that artificial intelligence (AI) may help in overcoming this challenge.
The study by researchers from the McGill University and the Ludmer Centre for Neuroinformatics and Mental Health shows that analysis of blood samples—collected from patients ailing with diseases such as Alzheimer's—by AI, can predict and decode the progression of these diseases. In the future, this could enable doctors in providing customised and effective treatment to individual patients.
"This test could one day be used by doctors to evaluate patients and prescribe therapies tailored to their needs," said Yasser Iturria-Medina, first author of the study in a statement.
Analysing blood and brain samples
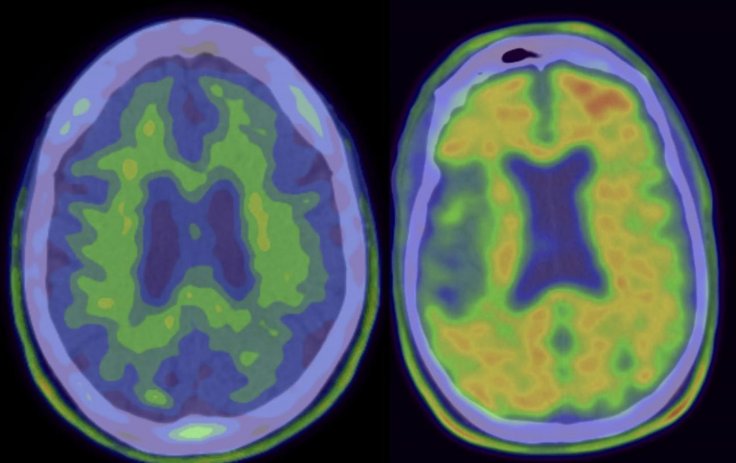
For the study, the researchers analysed the blood and post-mortem brain samples from 1969 patients who had been afflicted with Huntington's and Alzheimer's disease, using an AI algorithm. Their aim was to learn more about the molecular patterns that are specific to these diseases.
Detecting gene expression
The manner in which the patients' genes expressed themselves in distinct ways over the decades was detected by the algorithm. Neurodegenerative diseases such as Alzheimer's, progress over the years. Therefore, the detection of gene expression provided the first prolonged insight into the molecular changes central to neurodegeneration.
Earlier studies that focussed on neurodegeneration provided limited understanding about the characteristically slow progress of these diseases as they often employed 'snapshot' or static data. However, the current study concentrated on exposing the chronological information carried in expansive data by considering decades of disease development. This helped in uncovering the connection between the alterations in gene expression during that time and how the patients' condition changes due to it.
Molecular changes in brain and peripheral body are similar
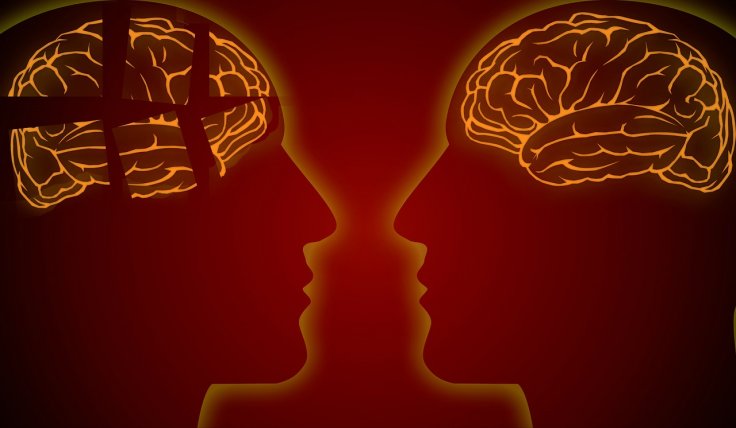
In addition to revelations from the brain samples, findings from the blood test led the scientists to conclude that there was a marked similarity between the molecular changes in the brain and the rest of the body. How did they arrive at this conclusion? The blood test was able to detect 85 to 90 percent of the main predictive molecular pathways that the test of post-mortem brain data also detected.
These findings may help in providing individualised treatment in the future. Also, it may be able to sort patients based on the level of progression of their conditions during clinical trials of experimental drugs. "It could also be used in clinical trials to categorize patients and better determine how experimental drugs impact their predicted disease progression," added Iturria-Medina
Growing integration of AI with health care
This study adds to the growing evidence supporting the effectiveness of integrating AI with medicine to treat various conditions. For example, a recent study by researchers from New York University's Langone Health and School of Medicine demonstrated that combining AI with advanced optical imaging produced precise and real-time intraoperative(during surgery) diagnosis of brain tumours. The AI-based image classification method was found to be more accurate in comparison to the interpretation of traditional histologic images by qualified pathologists.
Another study by researchers from National Health Service, Britain, Google Health, and Imperial College London found the Google's AI could detect breast cancer by screening mammograms with the same level of accuracy as professional radiologists. It was also found that the AI could reduce false-negative errors.