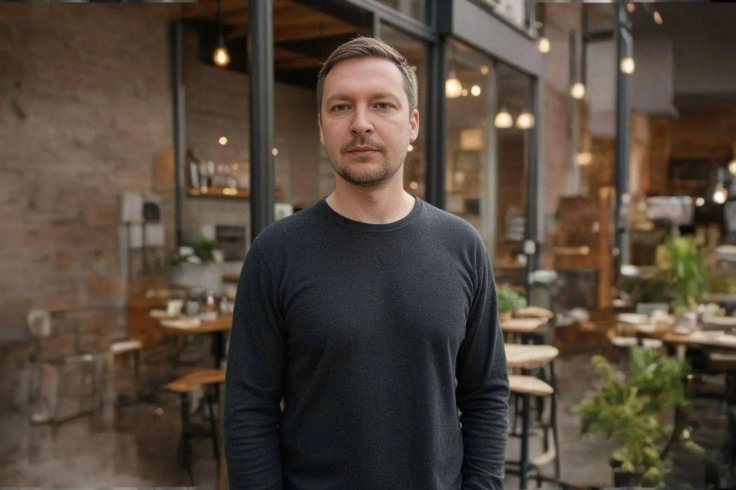
Given the magnitude of the impact of machine learning on practically every economic sector, one of the most intriguing topics becomes the specifics of the way data science, algorithms, and machine learning are impacting financial markets. A rising star and one of the most promising technologies in terms of portfolio optimization is Quant trading — an approach and technology that optimizes strategies by letting computers process and analyze large-scale datasets.
To see how data and machine learning are impacting the future of trading, we've talked to Andrey Kustarev, who currently holds the position of a Director of Portfolio Management at WorldQuant, one of the world's largest quantitative investment firms, managing approximately $9 billion in assets. With a strong background in both finance and technology, Andrey has been instrumental in running US equities systematic books combined with own signals. This outstanding career journey is backed by years in academia, since Andrey holds a Ph.D. in Mathematics from Moscow State University.
To start off, could you describe the general approaches to portfolio management and explain how Quant Trading fits into them?
First of all, portfolio management fundamentally deals with asset allocation, diversification, risk management, and performance tracking, each designed to optimize returns for a given risk level tailored to an investor's profile.
Quant trading, or quantitative trading, enhances these traditional methods by employing advanced mathematical models. It leverages large datasets, develops predictive models, and executes trades with unmatched precision and speed, and is particularly good at adapting to market changes. So, quant trading systematically uses complex market patterns which often lie beyond the scope of manual strategies.
You can imagine quant trading in portfolio management as the autopilot system in an aircraft. Just as autopilot uses a complex array of sensors, data inputs, and algorithms to navigate an airplane safely, efficiently, and often more effectively than a human pilot could under normal flying conditions, quant trading employs advanced mathematical models and computational power to navigate financial markets.
Who primarily uses Quant Trading technologies, and how widely is it adopted in the financial sector?
Right now institutional investors, hedge funds, proprietary trading firms, and investment banks are the main users. The adoption of these systems is more widespread than you might think, especially among elite financial firms who use this technology to gain a competitive edge in the current landscape. It might come as a surprise, but there are companies that have leveraged the power of quant trading for decades — DE Shaw и Renaissance Technologies to name a few.
Since the 1980's, these firms have made a fortune and become wildly successful players in the market.
Yet, back then only a select few went with this route, and the majority has started to catch up in the last 10 to 15 years. In this time we have seen a marked increase in use of quant trading, which is a clear reflection of broader trends in the industry towards automation and data-driven decision-making, with notable users including, Jane Street, Citadel, Two Sigma Investments, and WorldQuant — some among many.
Can you talk more about what impact has data science already had on financial markets, and what future changes could algorithms bring to fintech?
The magnitude of the impact is yet to be seen, but the changes data science has brought to the financial market is already profound enough. The main factors have become enhanced risk assessments and predictive analytics, which paved the way for more sophisticated automated trading systems. The future is unclear, but I am confident that progress in things such as deep learning will further refine market predictions, while more advanced algorithms might introduce personalized financial products and dynamic, real-time risk management, boosting both the accessibility and effectiveness of financial strategies.
Can you discuss how AI and machine learning are furthering portfolio optimization?
I mean, AI and machine learning have done unimaginable things for quant trading. This progress became possible thanks to complex predictive models that analyze market data in real-time. With things such as deep learning, these models can process decades of market data within minutes to predict trends with incredibly high accuracy, which in turn improves trade execution performance. Yet there are risks, which are important to keep in mind.
How extensively are AI and machine learning being adopted in trading environments? Who are some of the key players?
The adoption of AI and machine learning in trading has been growing, particularly in the last decade. This is facilitated by increased computational power and data availability. Key players include Renaissance Technologies, Two Sigma Investments, Citadel, DE Shaw, and WorldQuant, Cubist Systematic, DRW, SIG, Jane Street, XTX, Jump Trading — each known for their sophisticated quantitative and algorithmic trading approaches.
What are some of the major challenges financial institutions face when integrating advanced technologies into existing systems?
Integrating AI and machine learning into existing systems presents substantial challenges, something along the lines of refurbishing an old building with modern technology. Many financial institutions operate on legacy systems which have stood the test of time but are not easily compatible with the latest AI technologies, making upgrades quite costly. Moreover, effective AI applications rely on high-quality, well-organized data, but many institutions struggle with data that is scattered or siloed, requiring significant efforts to make it usable.
What emerging trends in AI and quant trading do you find most promising?
I believe the most promising trends in AI and quantum trading include advancements in reinforcement learning and natural language processing, which are rapidly enhancing decision-making capabilities and the analysis of unstructured data like news and social media.
And finally, a question that's been on the minds of many within the industry: What impact do you foresee AI and quant trading having on employment within the financial sector?
Finance is one of the sectors most significantly impacted by AI. Such as Goldman Sachs and JPMorgan Chase have some of the highest percentages of their payrolls likely to be disrupted by generative AI.
As these technologies become more advanced, we can expect a shift in the types of jobs available and the skills required to get those jobs. On one hand, automation and algorithmic trading are likely to reduce the need for traditional trading roles and some types of quantitative analysts, as computers can perform complex calculations and transactions faster and more accurately than we can.
On the other hand, there will be a growing demand for professionals in AI and data science. These roles will focus on developing, fine-tuning, and managing AI systems and strategies. Overall, while AI and quant trading may displace some jobs, they will also create new opportunities, best suited for those who choose to adapt to the changing landscape by gaining technological proficiency.