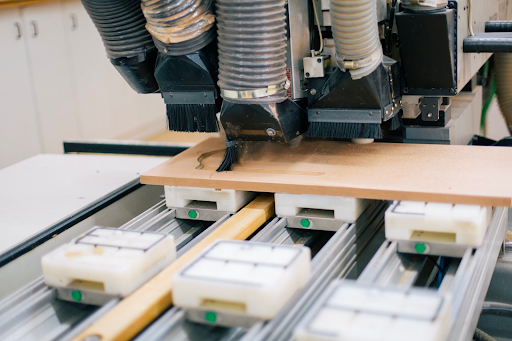
Pivotal advancements occur in marketplaces daily, such as data-centric artificial intelligence (AI) and the development of reliable and scalable platforms. Marketplaces seamlessly integrate with social media platforms, allowing users to purchase directly from their social media feeds. Additionally, the emergence of niche marketplaces tailored to specific needs and interests is a testament to their adaptability. In this expansion, the emphasis on elevating customer experiences through personalized product recommendations and efficient delivery options has become paramount.
The field of data-centric AI is undergoing a renaissance. New machine learning (ML) algorithms are surfacing, offering enhanced efficiency and accuracy compared to traditional counterparts. Ensuring fairness, predictability, and reliability in algorithms is more critical than ever.
With an illustrious career spanning DoorDash, Uber Technologies, Goldman Sachs, and JPMorgan Chase, Gayatri Iyengar is not just at the forefront but leading the charge in these technological advancements. Her insights are more than just observations; they culminate in a rich tapestry of experience, innovation, and leadership.
In an exclusive interview with International Business Times Singapore, Gayatri Iyengar shares her expert insights, shedding light on data-centric AI-driven marketplaces, real-time adaptability, enhancing user experiences, the science of operational efficiency, and predictability and reliability in today's rapidly changing technological world.
Welcome to IBT SG, Gayatri. Can you tell us a little about your experience, particularly in the marketplace, Infrastructure, and logistics?
Thank you for having me. I have more than 17+ years of experience in the technology industry. In my current role, I lead the Logistics and Dasher Growth Engineering organizations at DoorDash. I am responsible for spearheading core AI functions, including onboarding of dashers to our platform, forecasting real-time supply and demand, developing core matching algorithms, vehicle routing problems, delivery time predictions, pricing, generating optimal routes for our dashers, and, most importantly building a scalable, reliable fulfillment platform that redefines the future of labor. The products and platforms we build are the brains of DoorDash's operation and underpin its entire operational framework.
Before DoorDash, I bootstrapped and built the marketplace reliability platforms and observability organizations at Uber. My teams made Uber's observability stack, encompassing metrics, logs, traces, monitoring, alerting, and a comprehensive observability Application Performance Management (APM) solution. Before that, I had much experience building financial marketplaces, electronic and algorithmic trading at Goldman Sachs, and creating large data lakes and mobile apps at JPMorgan Chase.
Beyond my primary roles, I've derived immense satisfaction from nurturing the next generation of tech professionals. I extend my influence through advisory roles for multiple tech companies. I share insights at renowned conferences such as Women Impact Tech, CTO Connections, Lesbians Who Tech, Developer Week, DevCon, Google Women in Tech, TedConferences, and Grace Hopper.
Gayatri, you have experience serving as the head of engineering for logistics. What are some trends you see in building marketplaces and logistics?
Marketplaces and logistics are rapidly evolving sectors with an intense focus on innovation. The integration of commerce and social media platforms is happening at an unprecedented pace. Marketplaces leverage AI and ML to create personalized shopping experiences by recommending products, offering discounts, and providing relevant information. DoorDash, for instance, customized food delivery through AI, tailoring restaurant and dish recommendations based on user preferences.
In 2023, we shifted towards more transparent and explainable algorithms. The industry has recognized the importance of understanding how algorithms make decisions, especially in sectors with significant real-world implications. Additionally, there's a growing emphasis on real-time adaptability, allowing algorithms to adjust to changing data swiftly.
Additionally, these marketplaces are enhancing the shopping experience across various channels and introducing subscription services like DoorDash's DashPass, reducing delivery fees. Consumers expect speedy deliveries, pushing logistics providers to develop innovative last-mile delivery solutions.
Let's discuss best practices. With your years of professional experience, what are the key steps to maximize the efficiency of new algorithms?
- Firstly, it's crucial to understand the problem you're trying to solve clearly. Ensure your data is clean, relevant, and unbiased, and choose the correct algorithm for the job. Consider factors like the nature of data, available computing resources, and problem complexity.
- Select or engineer relevant features that provide meaningful information to the algorithm. Carefully select parts that can lead to faster convergence and better results.
- Tune the algorithm's hyperparameters for optimal performance. Use regularization techniques like L1 or L2 to prevent overfitting, leading to more efficient and robust models.
- Regularly benchmark and profile your algorithms to identify bottlenecks or areas for improvement. Profiling tools can help pinpoint inefficiencies.
Regularly review and update algorithms to account for changing dynamics. And lastly, always prioritize user feedback; it's invaluable in refining and optimizing algorithms.
In the fast-paced world of AI/ML, how do you promote a culture of continuous learning, experimentation, and effective planning within your teams, especially when dealing with high uncertainty about project outcomes?
This is a great question and a timely one. Here's my rule of thumb:
- Encourage teams to embrace challenges and uncertainties as growth opportunities. Setbacks are part of the path to success.
- Create an environment for trying new ideas and approaches. Celebrate both successes and failures, promoting a culture of experimentation.
- Choose agile approaches over rigid plans for adaptable project management in AI/ML.
- Develop risk mitigation plans for uncertainty. Identify and address potential challenges in advance.
- Acknowledge and celebrate even small achievements to boost team morale and motivation.
Balancing innovation with practicality is a critical aspect of AI/ML projects. How do you strike the right balance between working on innovative ideas and addressing practical, real-world problems?
Absolutely! Finding the sweet spot between innovation and practicality is key for AI and ML projects. Let me break it down for you in a relatable way.
Imagine we're all about improving food delivery at DoorDash. We want to make it a personalized and speedy experience. So, we listen to our users, like knowing your favorite restaurants and food choices. This is where the innovation part comes in. We use AI to develop ideas, like fancy algorithms to suggest restaurants that match your taste. That's the innovation part, making things exciting and tailored just for you.
But we don't stop there. We set clear goals, like making sure your food arrives faster. That's where practicality kicks in. We focus on projects that have a significant impact, like finding the quickest routes for our delivery drivers. Innovation is great, but it has to make sense for you. So, when we test those new algorithms for route optimization, we want to get your food to you quicker and more reliably. That's the practical side of things.
Throughout this whole journey, we collected a ton of data. This data helps us make things better. Now, here's the secret sauce: we allocate resources wisely. Some go into innovative research, like predicting delivery times using AI. But we also ensure our app is super easy for you to use. That's the practical part again.
Our project list is a mix of innovative and practical projects. This way, we're ready to tackle real-world challenges while staying at the cutting edge of AI and ML.
You led Reliability Platforms at Uber. What is the importance of observability and the role AIOps play in marketplaces?
Observability was a critical part of our operations when I worked at Uber. My team at Uber bootstrapped and built the open-source time series database M3 and Jaeger for tracing and Clickhouse-based inhouse logging platform. Imagine the bustling Uber marketplace, where millions of drivers and riders engage daily. Observability allows us to monitor everything in real time. We collected data from various sources, including mobile apps, GPS devices, and payment systems, to track the entire system. It was like having a radar for drivers and riders, demand in specific areas, and app performance.
Observability acted as our early warning system. If there was a sudden surge in ride cancellations or the app experienced downtime, it was our superhero sense tingling, alerting us to the issue so we could act swiftly and maintain a seamless user experience. To take this one step further, AIOps uses super-smart algorithms to make sense of all the data that Observability gathers. We built AI-based anomaly detection platforms that would auto-tune thresholds for microservices. This meant predicting problems before they happen, taking action, and fixing issues automatically.
But observability wasn't just about troubleshooting; it was our secret weapon for optimizing the marketplace. We could make data-driven decisions by analyzing data on driver behavior, rider preferences, and traffic patterns. For example, we could adjust prices on the fly to balance supply and demand, ensuring shorter wait times for riders and higher earnings for drivers. Collecting and analyzing a wealth of data, we gained insights into user behavior, preferences, and trends. For instance, we could use observability to understand when and where surge pricing was likely to occur, helping drivers and riders plan accordingly.
How are you using AI in logistics, and what does the future hold?
In building a marketplace, we used AI to make things run smoother and give our customers the best experience possible. When delivering your favorite meal, we use AI to determine the best route for our drivers. It's like solving a puzzle to find the shortest and quickest path to your door. This helps us get your food to you fast and hot. We also use AI to predict how much food you and others will order.
Have you ever wondered how we can tell you when your food will arrive? Well, that's AI, too. We use deep neural networks to predict when your order will arrive at your doorstep accurately. We're excited about even more incredible things AI can do. Imagine self-driving delivery vehicles or drones bringing your food. That's the kind of future we're working on, a future of improving our customers' experience faster and more reliably.
However, we must remember that algorithms and automation are tools; the human touch and creativity drive innovation.